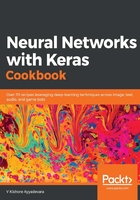
How to do it...
We'll code up the strategy defined previously as follows (please refer to the Categorizing news articles into topics.ipynb file in GitHub while implementing the code):
- Import the dataset :
from keras.datasets import reuters
(train_data, train_labels), (test_data, test_labels) = reuters.load_data(num_words=10000)
In the preceding code snippet, we loaded data from the reuters dataset that is available in Keras. Additionally, we consider only the 10000 most frequent words in the dataset.
- Inspect the dataset:
train_data[0]
A sample of the loaded training dataset is as follows:

Note that the numbers in the preceding output represent the index of words that are present in the output.
- We can extract the index of values as follows:
word_index = reuters.get_word_index()
- Vectorize the input. We will convert the text into a vector in the following way:
- One-hot-encode the input words—resulting in a total of 10000 columns in the input dataset.
- If a word is present in the given text, the column corresponding to the word index shall have a value of one and every other column shall have a value of zero.
- Repeat the preceding step for all the unique words in a text. If a text has two unique words, there will be a total of two columns that have a value of one, and every other column will have a value of zero:
import numpy as np
def vectorize_sequences(sequences, dimension=10000):
results = np.zeros((len(sequences), dimension))
for i, sequence in enumerate(sequences):
results[i, sequence] = 1.
return results
In the preceding function, we initialized a variable that is a zero matrix and imputed it with a value of one, based on the index values present in the input sequence.
In the following code, we are converting the words into IDs.
x_train = vectorize_sequences(train_data)
x_test = vectorize_sequences(test_data)
- One-hot-encode the output:
from keras.utils.np_utils import to_categorical
one_hot_train_labels = to_categorical(train_labels)
one_hot_test_labels = to_categorical(test_labels)
The preceding code converts each output label into a vector that is 46 in length, where one of the 46 values is one and the rest are zero, depending on the label's index value.
- Define the model and compile it:
from keras.models import Sequential
from keras.layers import Dense
model = Sequential()
model.add(Dense(64, activation='relu', input_shape=(10000,)))
model.add(Dense(64, activation='relu'))
model.add(Dense(46, activation='softmax'))
model.summary()
model.compile(optimizer='adam',loss='categorical_crossentropy',metrics=['accuracy'])

Note that while compiling, we defined loss as categorical_crossentropy as the output in this case is categorical (multiple classes in output).
- Fit the model:
history = model.fit(X_train, y_train,epochs=20,batch_size=512,validation_data=(X_test, y_test))
The preceding code results in a model that has 80% accuracy in classifying the input text into the right topic, as follows:
